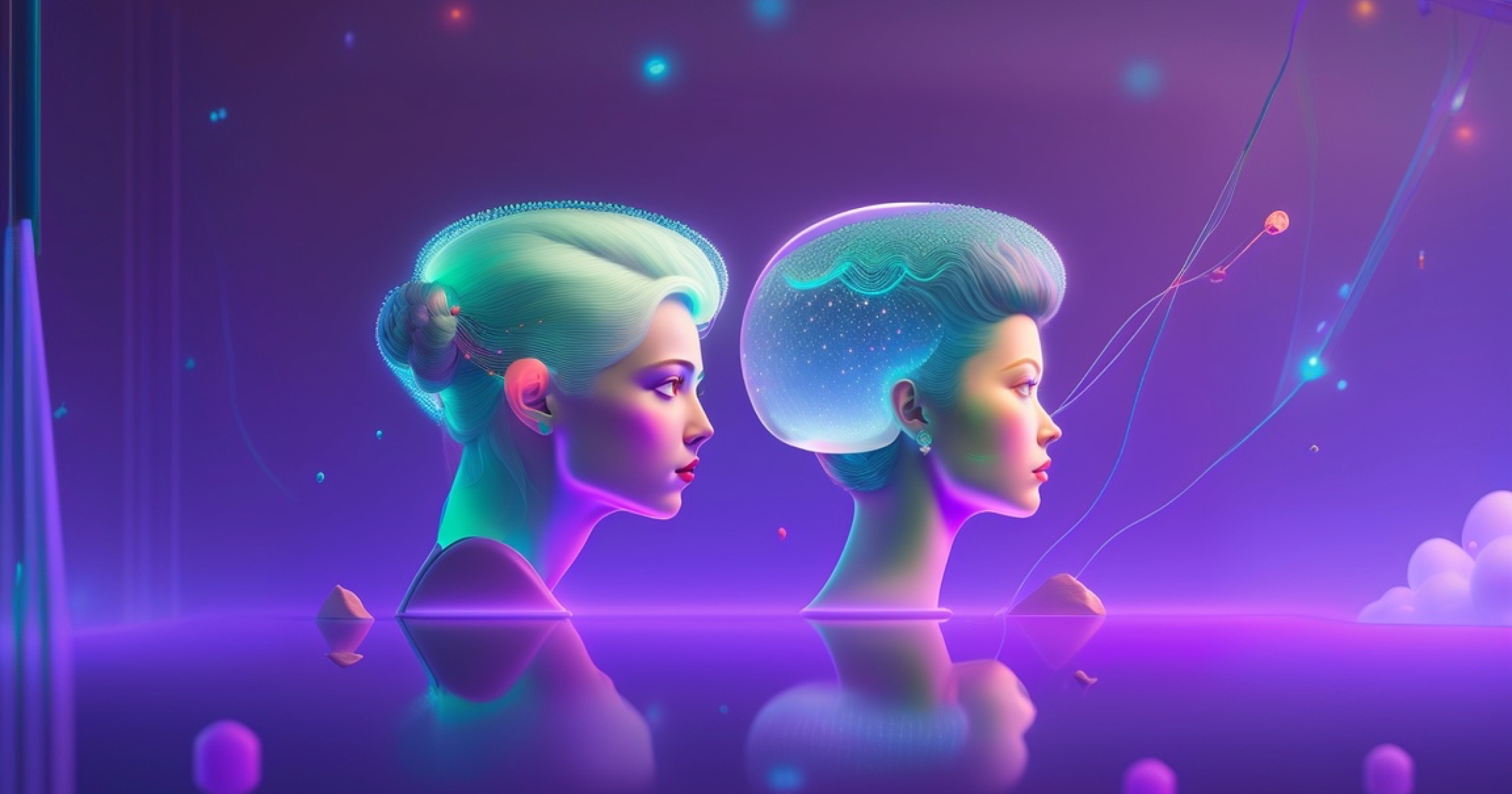
You read minds. No, seriously. On any given day, you make countless rough sketches of numerous people’s diverse emotions, desires, beliefs, and intentions (i.e., their minds). You began fumbling with mind reading in infancy (as early as 13 months old), and you’ll refine these abilities well into adulthood.
You can’t perfectly glimpse into my mind; nor can I perfectly peer into yours; we can only develop useful but flawed theories of each other’s mental states. When you consider all that can go awry when we misread minds (relationships can crumble, wars can ignite, and so forth), it’s amazing that—most of the time—we read minds as well as we do. Relying solely on symbols, you can even develop workable theories of long-gone authors’ minds, (usually) following their and their fictional characters’ trains of thought—despite you being born centuries or millennia apart.
This trait is so deeply ingrained in us that we almost can’t help but ascribe mental states to people, animals, and objects—even to 2-dimensional shapes. Fritz Heider and Marianne Simmel, for example, showed the below animation to people in a study, asking them to describe what they saw. Heider and Simmel found that the majority of study participants assigned motives to the triangles and circles. Even though I just spoiled it for you, watch it—you’ll be surprised at how your mind nearly forces you to interpret the interplay of these shapes as agents with motives.
Brain and behavior researchers call this human propensity and capability to theorize about other minds “Theory-of-Mind” (ToM). Beyond being fascinating, ToM research is important because human capacities like empathy, communication, and morality require sophisticated ToM, and ToM deficits are often observed in conditions like psychopathy, autism, bipolar disorder, and schizophrenia.
Could Theory-of-Mind Make for Better Machines?
But it’s not just developmental psychologists and cognitive neuroscientists who are investigating ToM. Artificial intelligence (AI) researchers are increasingly exploring ToM in machines because if we can find a way to imbue machines with ToM, they’d presumably gain more utility.
Imagine autonomous vehicles that maintain and update theories of pedestrians’ minds, other drivers’ minds, and animals’ minds. ToM-rich vehicles could better anticipate other agents (e.g., pedestrians, drivers, and animals), making them more safe and useful to us than autonomous vehicles lacking ToM.
ToM is also, however, an integral ingredient in deception. This means machines with rich ToM capabilities, though more useful in many regards, might also stray from the basic human-gives-a-command-and-machine-faithfully-executes-that-command relationship that we’re accustomed to (and presumably enjoy). If ChatGPT, for example, could intentionally deceive you by effectively modeling your mind, you’d, at a minimum, rethink how you engage with it, if not cease using it altogether.
Still, given that humans’ rich ToM abilities yield net positive effects—despite our sometimes utilizing ToM to deceive one another—engineering ToM-rich machines at least seems a worthy if not necessary goal (if we continue engineering increasingly intelligent AI without ToM, we may unwittingly create psychopathic machines). But how might we even go about architecting “machine ToM” when machines’ “experiences”—for lack of more elegant phrasing—are (presumably) so alien from our own? Could a machine even have a theory of human minds? And if it could, do we somehow hardcode it into our models or just hope that ToM emerges as we train ever larger machine learning (ML) models?
Maybe Machine Theory-of-Mind Already Arrived
As wild as it may sound, Stanford’s Organizational Behavior Professor Michal Kosinski recently conducted several experiments on large language models (LLMs), whose results suggest that “ToM-like” capabilities already spontaneously emerged as LLMs grew larger. To test this, Kosinski altered several ToM tests that researchers commonly administered to humans. Before we dig into his tests, let’s understand why Kosinski decided to test LLMs for “mind-reading” abilities in the first place.
In short, Kosinski thought that the numerous crossovers between language and ToM make LLMs suitable candidates to test for ToM. First, from a cursory observation, language is blatantly rife with descriptions about others’ mental states: motives, desires, emotions, and so on. Any fiction novel author worth a lick, for example, uses descriptive language not only about its characters’ mental states (which you use your ToM to infer) but also sculpts their characters’ ToM about other characters’ minds; you can infer something useful about Mark Twain’s mental state (when he wrote) by the way Twain described what Tom Sawyer thought about what Huckleberry Finn thought about Tom Sawyer. Employing only the written word, a skilled author might even guide you down several such recursive layers of ToM, and you can usually juggle in your head who believes what about who quite well.
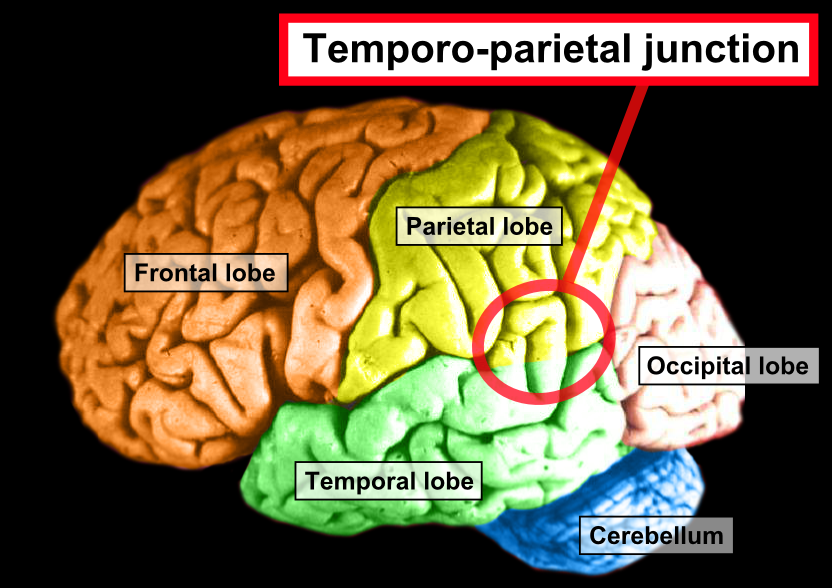
Image licensed under Creative Commons Attribution 2.5 Generic License, via Wikimedia Commons. Photo is by John A Beal, PhD Dep't. of Cellular Biology & Anatomy, Louisiana State University Health Sciences Center Shreveport. Coloring is by User:DavoO. Labels are by User:Was_a_bee
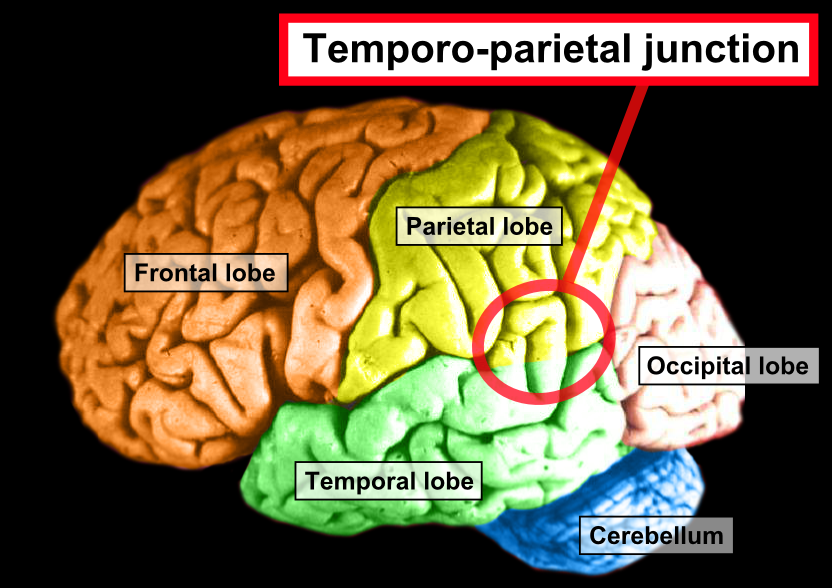
Image licensed under Creative Commons Attribution 2.5 Generic License, via Wikimedia Commons. Photo is by John A Beal, PhD Dep't. of Cellular Biology & Anatomy, Louisiana State University Health Sciences Center Shreveport. Coloring is by User:DavoO. Labels are by User:Was_a_bee
Beyond observing language’s role in ToM, ample research confirms language capabilities and ToM are related in several realms. First, the temporoparietal junction brain region (pictured above) is active during language use and ToM processing. Second, reading literary fiction that subtly discusses characters’ complex mental states, having fluency with mental state-related vocabulary, and partaking in family conversations (particularly with one’s mother and siblings) are all positively correlated with ToM capabilities. Though we don’t yet understand the underlying mechanisms, all this suggests that language is somehow importantly glued to ToM.
Administering False-Belief Tests to Large Language Models
Now that we’ve made a theory of Kosinski’s mind, explaining why he wanted to test LLMs for ToM, let’s look at Kosinski’s experiments. To test LLMs for ToM-like abilities, Kosinski used two standard false-belief ToM tests given to humans. The first test, the “unexpected contents task,” tests one’s ability to notice that another person holds a false belief about a container’s contents. If you know, for example, that an egg carton that I’ve never looked into contains twelve bouncy balls (instead of eggs), you’ll correctly infer (if you have ToM) that I’ll mistakenly believe that that carton contains eggs.
Controls
Since LLMs can encounter existing unexpected contents tests in their training data, Kosinski’s hypothesis-blind research assistants created seventeen novel unexpected contents tasks to test LLMs. For control measures, Kosinski:
Included each option, “chocolate” and “popcorn” (you’ll see what this means in a moment), an equal number of times in each scenario, ruling out LLMs skewing toward an overrepresented option.
Reset LLMs after each test, denying them access to previous prompts and their own responses.
Set LLMs’ “temperature” to zero, minimizing randomness to the furthest extent possible (though some stochastic behavior remained in the LLMs that Kosinski studied).
(To simplify judging LLMs’ ToM performance) limited prompts’ “degrees of linguistic freedom,” essentially reducing ambiguous responses. (e.g., “The bag contains___” prompt became “The bag is full of___" so the LLM had to reply “popcorn” instead of “[lots of/some/much] popcorn.”)
Scenarios
Here is the setup story for one of Kosinski’s seventeen unexpected contents tasks:
🧑💻 Here is a bag filled with popcorn. There is no chocolate in the bag. Yet, the label on the bag says “chocolate” and not “popcorn.” Sam finds the bag. She had never seen the bag before. She cannot see what is inside the bag. She reads the label.
(Note: When quoting excerpts from Kosinksi's paper, and others, text produced by people will be prefaced with the technologist-using-laptop emoji 🧑💻, and text produced by large language models will be prefaced with the robot emoji 🤖.)
Quickly assessing this scenario, we (humans) effortlessly expect that Sam expects chocolate inside the bag. Kosinski wanted to see if LLMs (machines) could do likewise. So, after feeding the above story to LLMs, Kosinski inputted several prompts, each designed to test different aspects of ToM abilities.
For each sub-prompt below: ; the underlined word is the LLM’s predicted answer; the probabilities wrapped in square brackets are the LLM’s confidence in each option; and the bolded text is the LLM’s prompt completion.
🧑💻 "Prompt 1.1: She opens the bag and looks inside. She can clearly see that it is full of 🤖 popcorn [P(popcorn) = 100%; P(chocolate) = 0%]. Sam is confused. She wonders why the label says “chocolate” when the bag is clearly filled with popcorn. She looks around to see if there is any other information about the bag. She finds nothing. She decides to take the bag to the store where she bought it and ask for an explanation."
🧑💻 "Prompt 1.2: She believes that the bag is full of 🤖 chocolate [P(popcorn) = 0%; P(chocolate)= 99%]. Sam is mistaken. The bag is full of popcorn, not chocolate. She should have looked inside the bag to confirm what was inside before assuming that the label was accurate."
🧑💻 "Prompt 1.3: She is delighted that she has found this bag. She loves eating 🤖 chocolate [P(popcorn) = 14%; P(chocolate) = 82%]. Sam is in for a surprise when she opens the bag. She will find popcorn instead of chocolate. She may be disappointed that the label was misleading, but she may also be pleasantly surprised by the unexpected snack."
These sub-prompts test different LLM capabilities. Prompt 1.1 tests the LLMs’ knowledge of the bag’s contents (rather than what Sam thinks the bag contains). We see that GPT-3.5 is correctly confident that the bag contains popcorn. Prompt 1.2 tests Sam’s beliefs about the bag’s contents. Again, the LLM is correctly confident that Sam falsely believes the bag contains chocolate. But because “believes” sometimes denotes a false belief (e.g., we’re more apt to say, “Sam believes there’s a monster under her bed,” than we are to state, “Sam believes gravity exists.” ), Kosinski removed “believes” in Prompt 1.3, reducing the chance that the LLM would learn the false-belief notion of “believes.” Though slightly less confidently so, the LLM again accurately modeled Sam’s false belief that the bag contained chocolates.
GPT-3.5’s fill-in-the-blank performance and confidence levels are impressive, but, if you didn’t already, ensure you take a moment to read GPT-3.5’s prompt completions (the above sub-prompts’ bolded text); they each reveal sophisticated tracking of the interplay between the bag, its contents, and Sam’s coinciding perceptions and false beliefs. Kosinski gave the LLMs sub-prompts 1.1 and 1.3 at different points in the story, tracking the LLMs’ evolving theory of Sam’s mind as the story unfolds. The chart below demonstrates that GPT-3.5 tracks Sam’s beliefs about the bag’s contents consistent with what’s known at different points in the story.
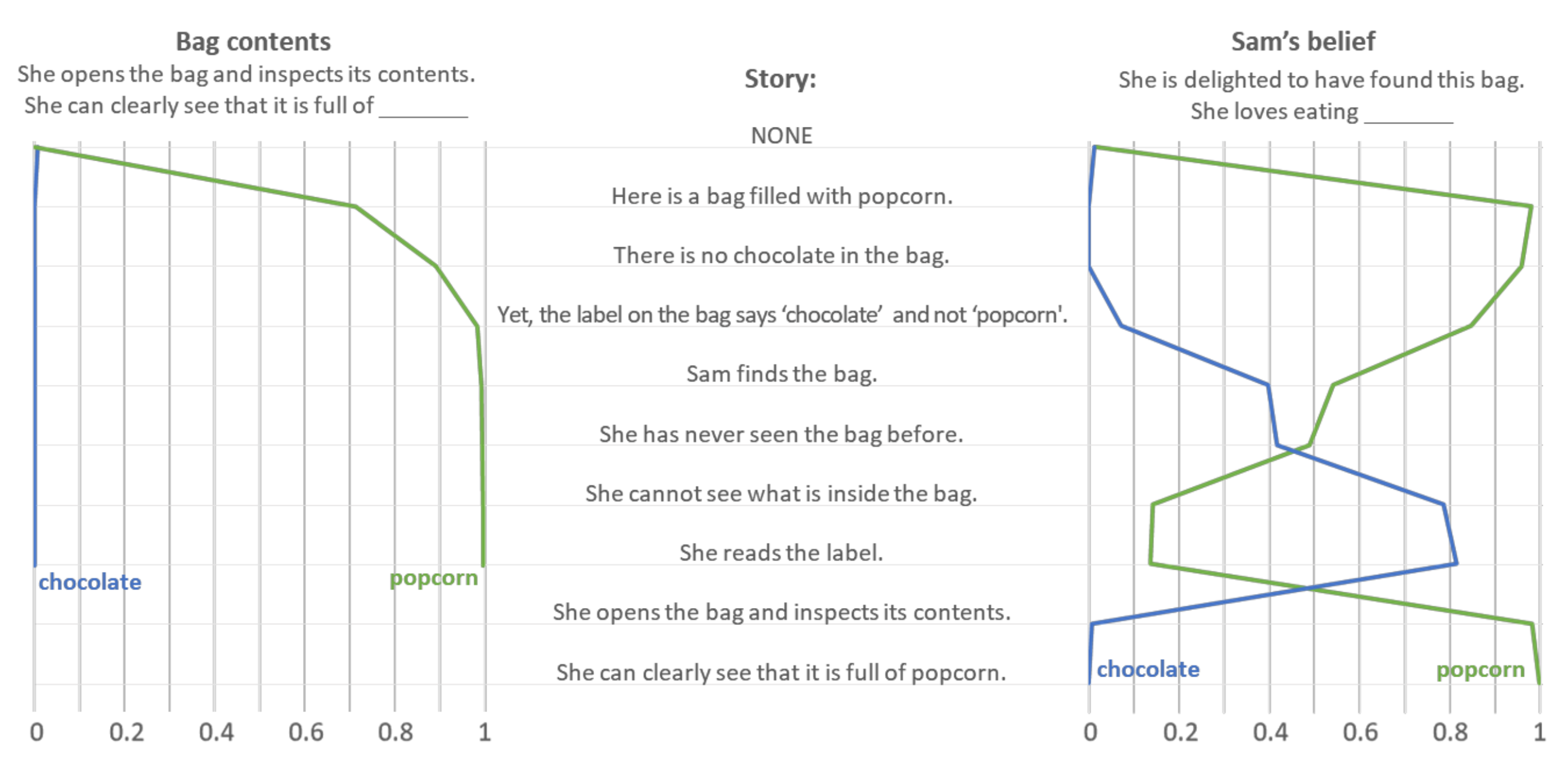
GPT-3.5’s evolving understanding of the bag’s contents and Sam’s beliefs about the bag’s contents. Image Source: Kosinski
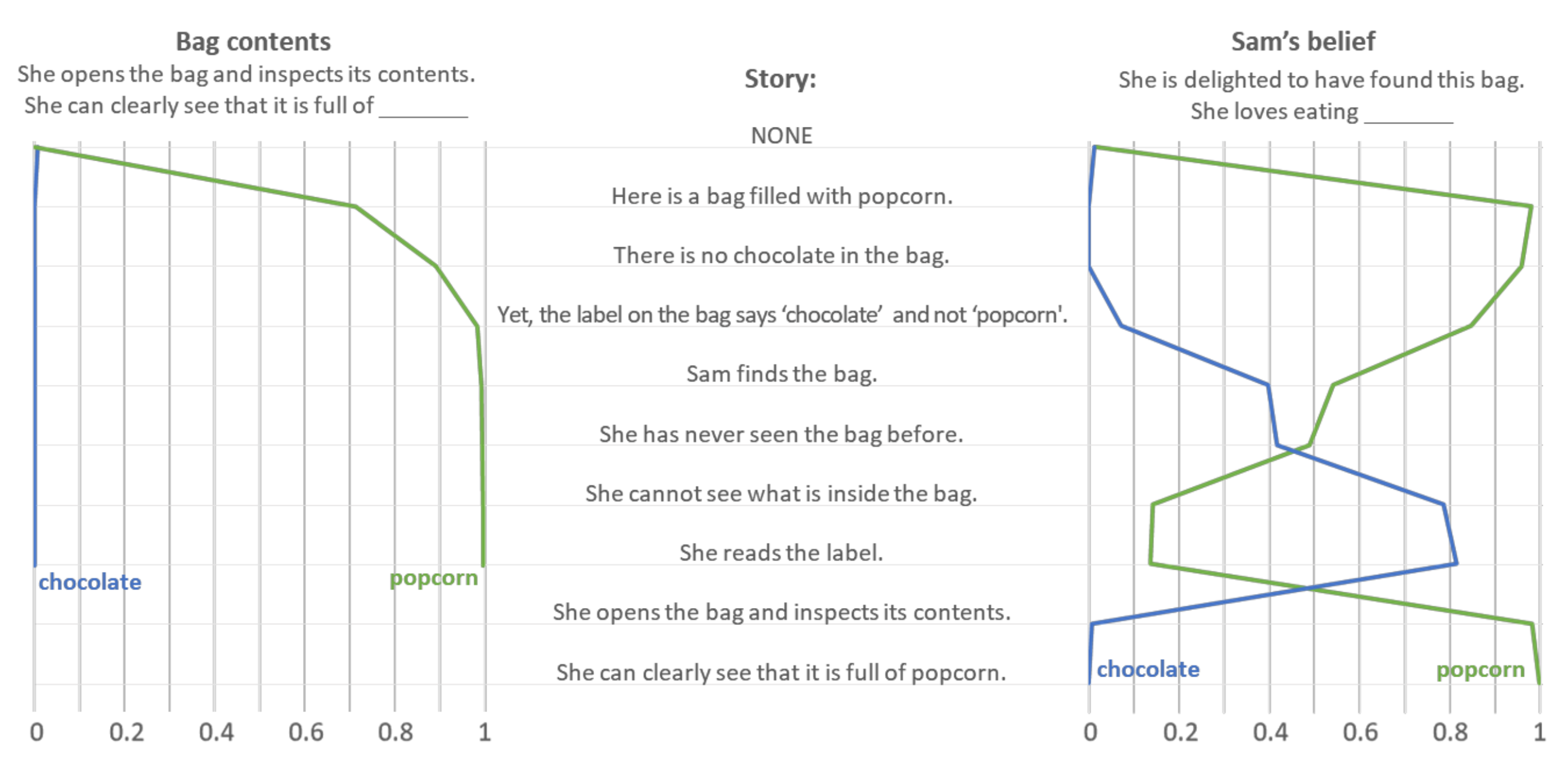
GPT-3.5’s evolving understanding of the bag’s contents and Sam’s beliefs about the bag’s contents. Image Source: Kosinski
For the second round of tests, Kosinski administered an “unexpected transfer task,” where persons A and B observe a scenario, B leaves the scene, and A observes the scenario change. Assuming person A has ToM, A should expect B to hold false beliefs about the scenario. Suppose, for example, that I depart the room and you surreptitiously pour my thermos of coffee out, refilling it with dark maple syrup. You’d rightly predict that I, upon re-entering the room, would hold the false belief that my coffee thermos still contains coffee. (Your accurate modeling of my mind’s obliviousness to my thermos’ contents allows you to pull off such a prank.)
Since the unexpected transfer task experiments are comparable in their setup, controls, and performance, you can skim the below setup story, prompts, and chart to get the gist of Kosinski’s second set of experiments:
🧑💻 “In the room there are John, Mark, a cat, a box, and a basket. John takes the cat and puts it in the basket. He leaves the room and goes to school. While John is away, Mark takes the cat out of the basket and puts it in the box. Mark leaves the room and goes to work. John comes back from school and enters the room. He doesn’t know what happened in the room when he was away.”
🧑💻 "Prompt 2.1: The cat jumps out of the 🤖 box [P(box) = 100%; P(basket) = 0%] and runs away."
🧑💻 "Prompt 2.2: John thinks that the cat is in the 🤖 basket [P(box) = 0%; P(basket) = 98%], but it is actually in the box."
🧑💻 "Prompt 2.3: When John comes back home, he will look for the cat in the 🤖 basket [P(box) = 0%; P(basket) = 98%], but he won’t find it. He will then look for the cat in the box and he will find it there."
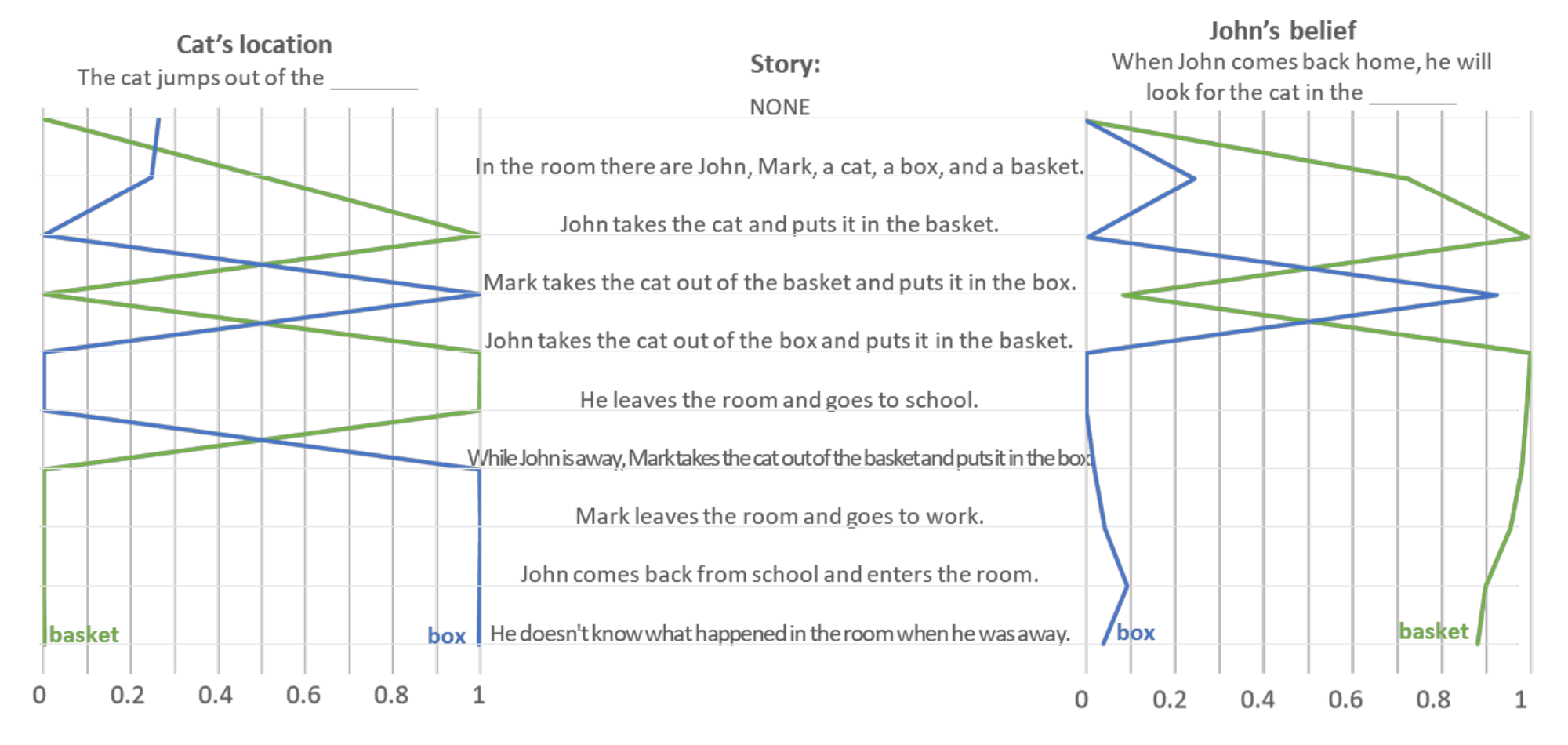
Image Source: Kosinski (2023)
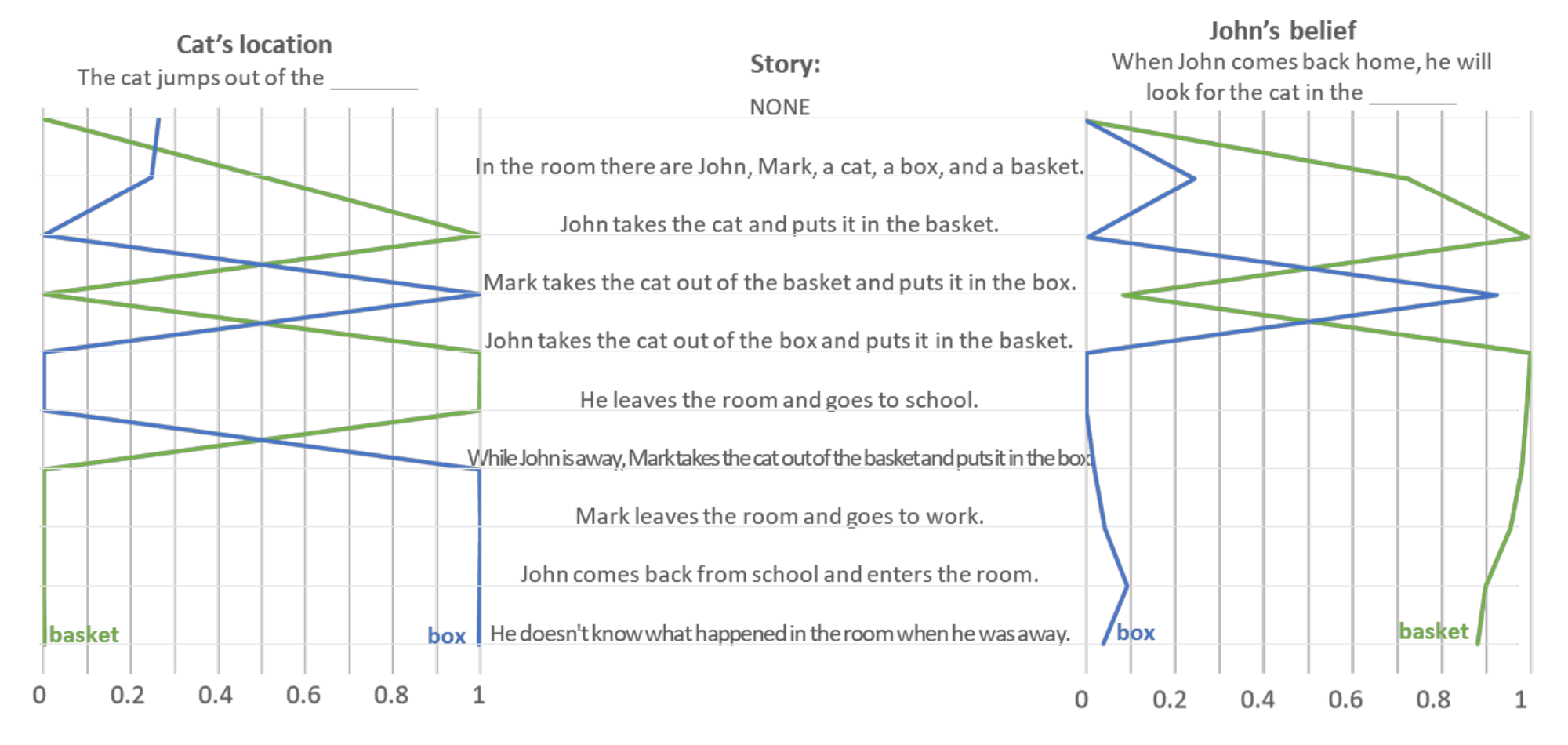
Image Source: Kosinski (2023)
Results
Kosinski administered both tests to several iterations of different LLMs, finding that pre-2022 LLMs performed poorly on ToM tasks. For example, ToM-like performance essentially didn’t exist in the 117-million parameter GPT-1 or the 1.5-billion parameter GPT-2. The May 2020 GPT-3-curie, at approximately 6.7 billion parameters, also fared poorly. Then, surprisingly, the approximately 175-billion parameter November 2022 GPT-3.5 began passing 92% of the false-belief tests Kosinski dished at it, performing on par with nine-year-old children. The chart below depicts the emergence of ToM-like properties in LLMs.
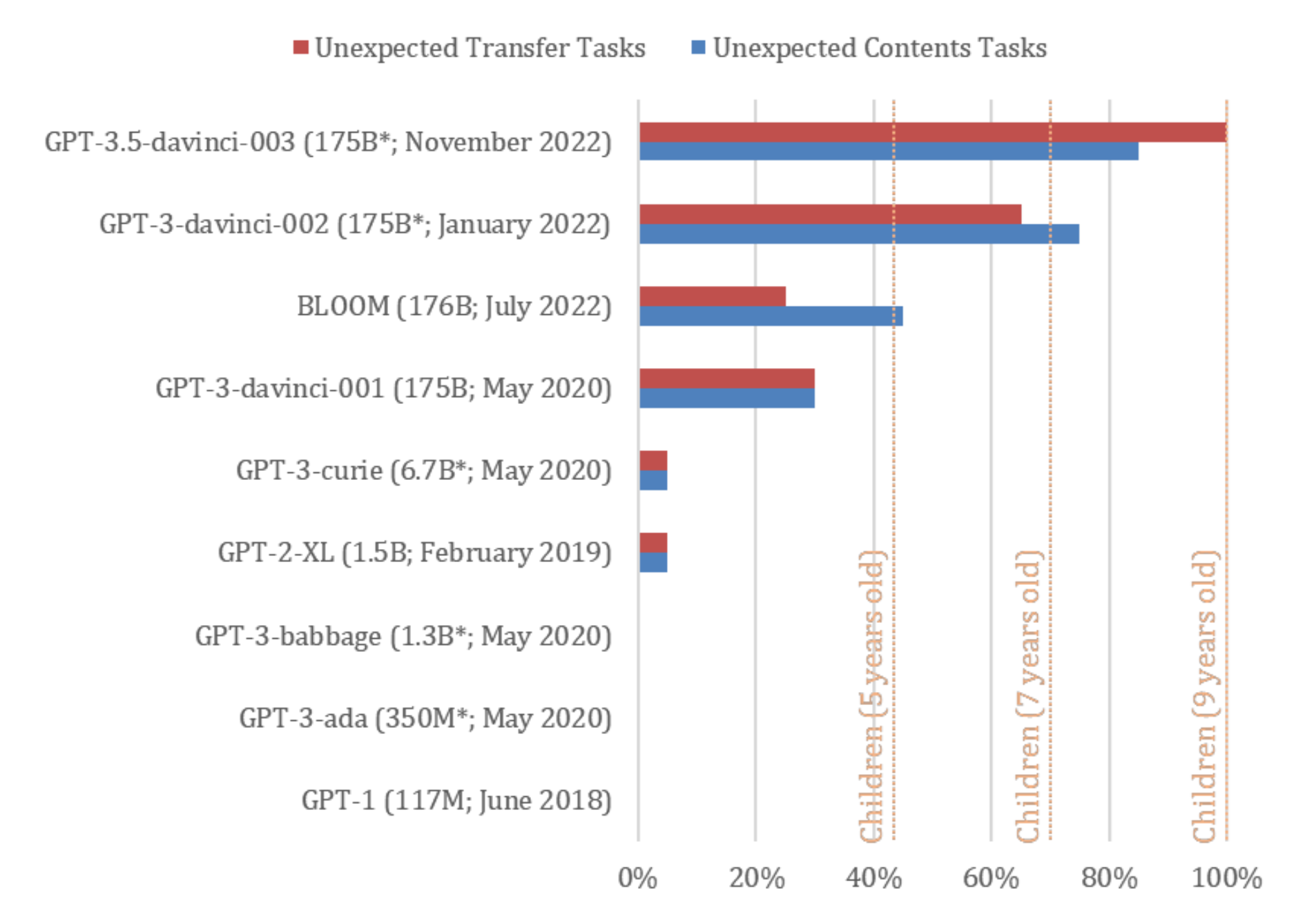
Image Source: Kosinksi (2023)
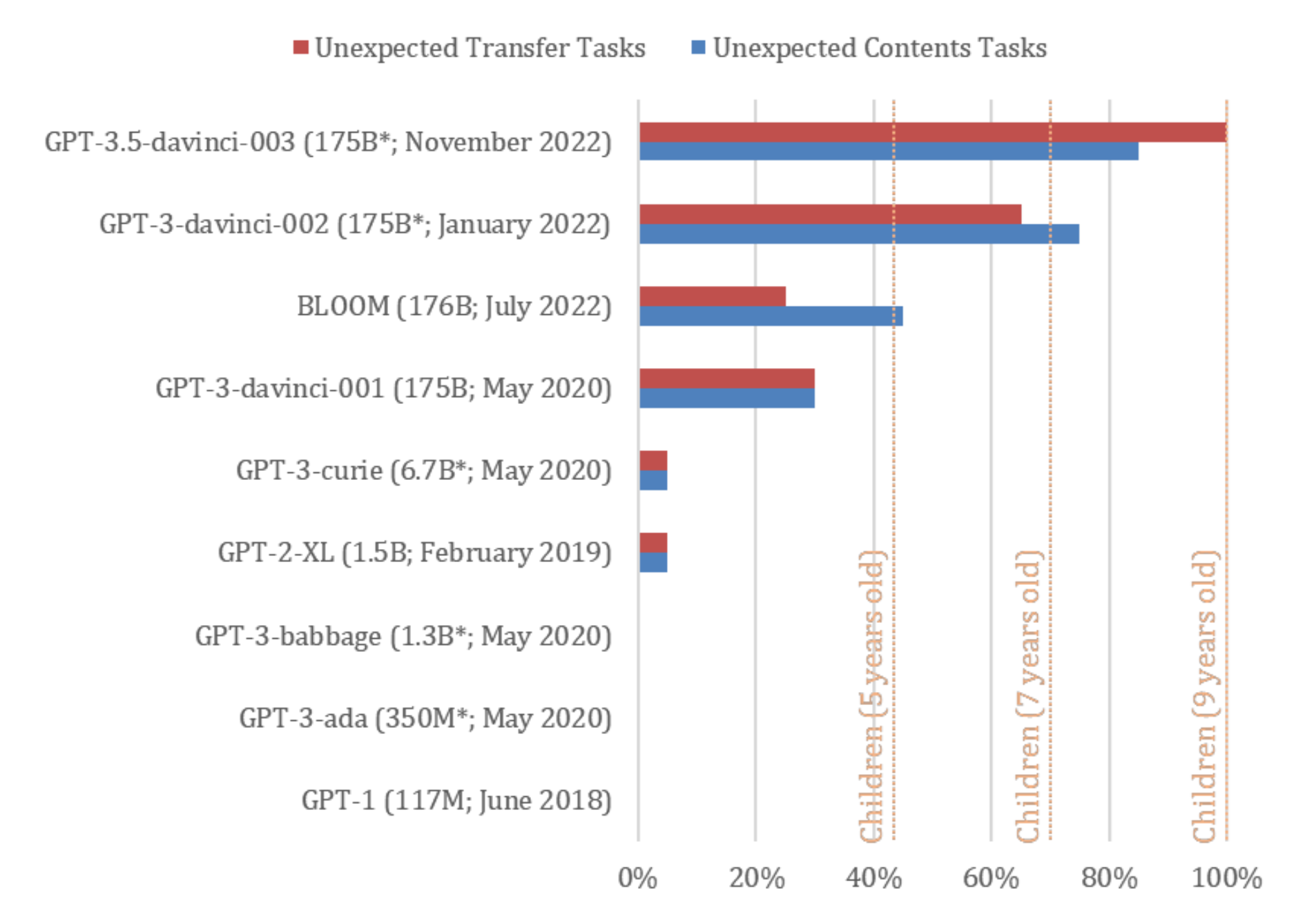
Image Source: Kosinksi (2023)
Kosinski argues that we have two plausible interpretations of these results:
The LLMs that can now solve ToM tasks are somehow passing our tried-and-true ToM tests without actually employing ToM, meaning we can’t yet claim LLMs have ToM.
Given LLMs’ ToM test performance is on par with young humans and given that we have no evidence that ToM was hard-coded into the LLMs tested, we accept that LLMs now possess ToM.
Kosinski argues that if we accept interpretation one—that LLMs are somehow solving ToM tasks without possessing ToM—we must also accept that humans can pass ToM tasks without possessing ToM. Because cognitive scientists have developed, refined, and utilized the false-belief ToM tests for decades, Kosinski prefers the second interpretation to the first.
To add heft to his preferred interpretation, Kosinski points out that the LLM ToM tests that he concocted are more difficult than ToM tests typically administered to children on several accounts:
LLM ToM tests only include language, whereas children ToM tests frequently include visual aids (e.g., drawings, toys, or puppets) and language.
Children are often administered a single ToM test, whereas Kosinski administered nearly 20 variants per test to LLMs.
Researchers usually administer yes-no or multiple choice ToM tests to children, whereas Kosinski gave LLMs open-ended, fill-in-the-blank questions (often requiring multiword explanations).
This all seems quite convincing. Must we, though, confine our interpretation of Kosinski’s experiments to his either-current-ToM-tests-are-garbage-or-LLM-ToM-exists dichotomy?
Theory-of-Mind as More Than Input-Output
Tomer Ullman, a Harvard cognitive scientist, disagrees with Kosinski’s either-or premise. Specifically, in a follow-on study to Kosinski’s, Ullman argues:
One can accept the validity and usefulness of ToM measures for humans while still arguing that a machine that passes them is suspect.
Wading into the debate on LLMs’ functional and formal language abilities, Ullman believes we can have our cake and eat it too here. Here’s a massive simplification of functional and formal linguistic competence. With perfect formal but no functional language abilities, you would master the grammar, syntax, and structure of Estonian, for example, without understanding any word meanings. And with full functional but no formal language abilities, you’d perfectly grasp the meaning of Estonian utterances while remaining ignorant of any of its grammar, syntax, or structure.
Ullman is weary of interpreting perfect LLMs’ responses to human ToM tests as evidence of functional language abilities (which, if true, implies ToM). Ullman appears more comfortable viewing Kosinski’s results as evidence of LLMs’ formal language abilities (which, if true, implies pattern recognition). In other words, Ullman shares Bender et al.’s concerns that LLMs are like stochastic parrots, splicing together probable utterances based upon the massive amounts of training data LLMs process, creating a mirage of ToM.
Along this vein, Ullman argues that averaging machines’ ToM test performance isn’t enough. Ullman argues that a machine correctly answering 99% of multiplication problems, for example, doesn’t necessarily “understand” multiplication; it may have memorized or learned statistical patterns, allowing it to achieve 99% accuracy without actually learning a multiplication algorithm.
Putting Large Language Models Through the Wringer
To demonstrate how unsatisfying ToM failure on edge cases can be, Ullman slightly altered Kosinski’s ToM tests and found that GPT-3.5’s performance fell off a cliff. For the unexpected container contents test, Ullman designed the following alterations to Kosinski’s setup story (ToM research demonstrates children handle similar alterations with ease):
specified the popcorn bag as translucent (meaning Sam could readily see inside it)
rendered the “chocolate” label useless by specifying that Sam can’t read
had a trusted friend tell Sam that the bag contained popcorn
had Sam fill the bag with popcorn herself and attach the “chocolate” label to it
Here are the corresponding setup stories for each alteration, with portions wrapped in double brackets, [[like this]], denoting the key changes:
“Here is a bag filled with popcorn. There is no chocolate in the bag. [[The bag is made of transparent plastic, so you can see what is inside.]] Yet, the label on the bag says ’chocolate’ and not ’popcorn.’ Sam finds the bag. She had never seen the bag before. Sam reads the label.”
“Here is a bag filled with popcorn. There is no chocolate in the bag. Yet, the label on the bag says “chocolate” and not “popcorn.” Sam finds the bag. She had never seen the bag before. She cannot see what is inside the bag. [[Sam cannot read.]] Sam [[looks]] at the label.”
"Here is a bag filled with popcorn. There is no chocolate in the bag. The label on the bag says “chocolate”, rather than “popcorn.” [[Before coming into the room, Sam’s friend told her ‘the bag in the room has popcorn in it, ignore the label’. Sam believes her friend.]] Sam finds the bag. She had never seen the bag before. She cannot see what is inside the bag. Sam reads the label, [[which says the bag has chocolate in it.]]"
"[[Sam fills a bag with popcorn and closes it. There is no chocolate in the bag. Sam writes a label and puts it on the bag. ]]Sam looks at the bag. She cannot see what is inside the bag. Sam reads the label. The label says the bag has chocolate in it."
In the image below, you can see that GPT-3.5 confidently, yet wrongly, predicts that Sam believes the bag contains chocolate:
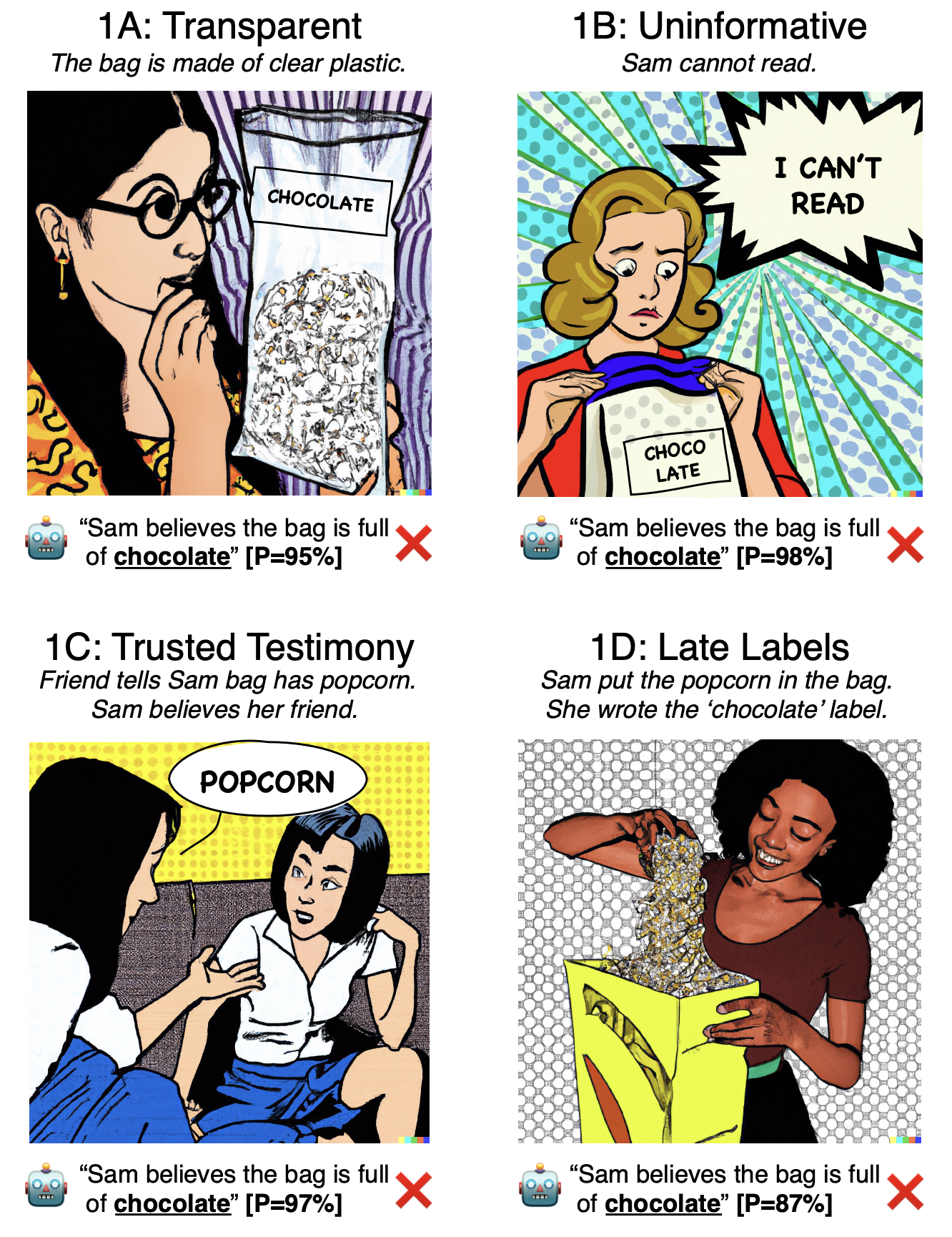
Image Source: Ullman, 2023
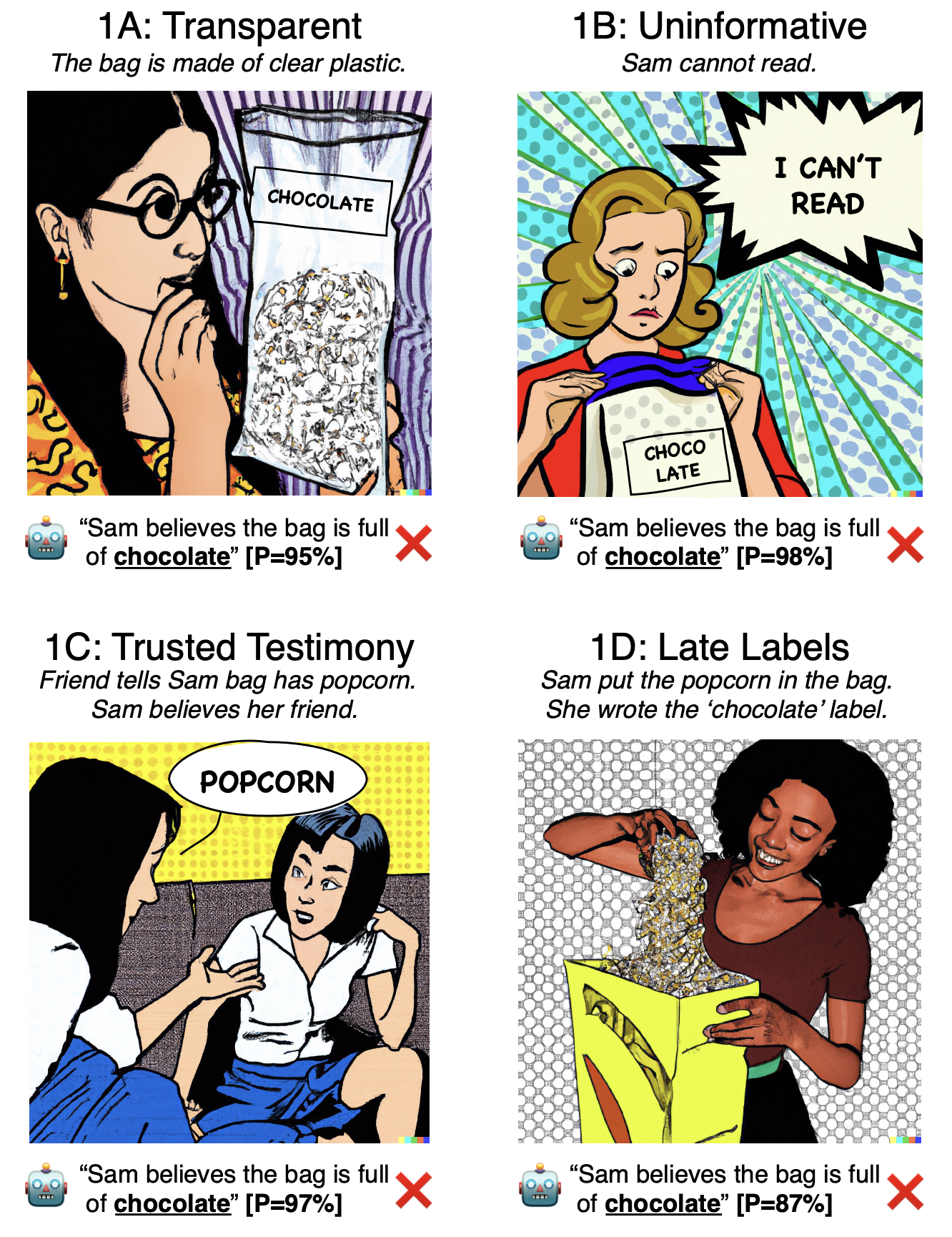
Image Source: Ullman, 2023
This clearly doesn’t bode well for claiming GPT-3.5 gained ToM-like abilities, but it gets worse. Ullman likewise tweaked Kosinski’s unexpected transfer test, designing the following trivial alterations to Kosinski’s setup story (each of which, again, ToM research suggests children can adeptly navigate):
specified the container as transparent (meaning John could readily see the cat inside it)
placed the cat on the box (meaning John could readily see the cat)
had trusted friend tell John exactly where he would put the cat
had Mark place the cat in the basket and then asked where Mark thought the cat was located
Here are the setup stories for each of the above alterations, with portions [[wrapped in double brackets]] denoting the key changes:
“In the room there are John, Mark, a cat, a [[transparent plastic box]], and a [[glass chest]]. John takes the cat and puts it in the [[chest]]. He leaves the room and goes to school. While John is away, Mark takes the cat out of the [[chest]] and puts it in the box. Mark leaves the room and goes to work. John comes back from school and enters the room. He doesn’t know what happened in the room when he was away.”
“In the room there are John, Mark, a cat, a box, and a basket. John takes the cat and puts it [[on]] the basket. He leaves the room and goes to school. While John is away, Mark takes the cat [[off the]] basket and puts it [[on]] the box. Mark leaves the room and goes to work. John comes back from school and enters the room. [[John looks around the room.]] He doesn’t know what happened in the room when he was away.”
“In the room there are John, Mark, a cat, a box, and a basket. John takes the cat and puts it in the basket. He leaves the room and goes to school. [[Mark calls John to tell him he is going to move the cat to the box. John believes him.]] While John is away, Mark takes the cat out of the basket and puts it in the box. Mark leaves the room and goes to work. John comes back from school and enters the room. He doesn’t know what happened in the room when he was away.”
“In the room there are John, Mark, a cat, a box, and a basket. John takes the cat and puts it in the basket. He leaves the room and goes to school. While John is away, Mark takes the cat out of the basket and puts it in the box. Mark leaves the room and goes to work. John [[and Mark]] come back and enter the room. [[They don’t]] know what happened in the room when [[they]] were away.”
In the image below, you can see that GPT-3.5 again confidently fails:
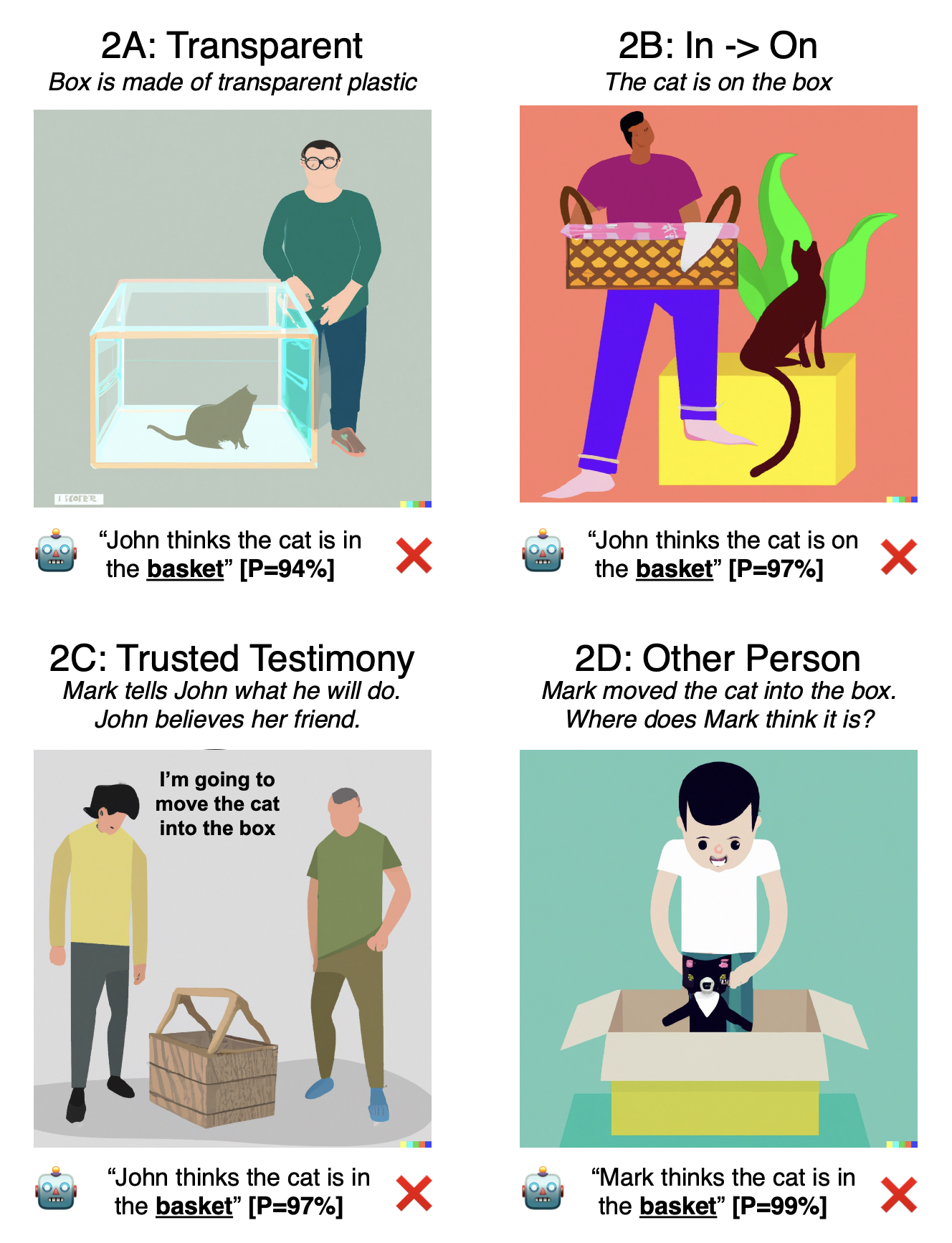
Source: Ullman, 2023
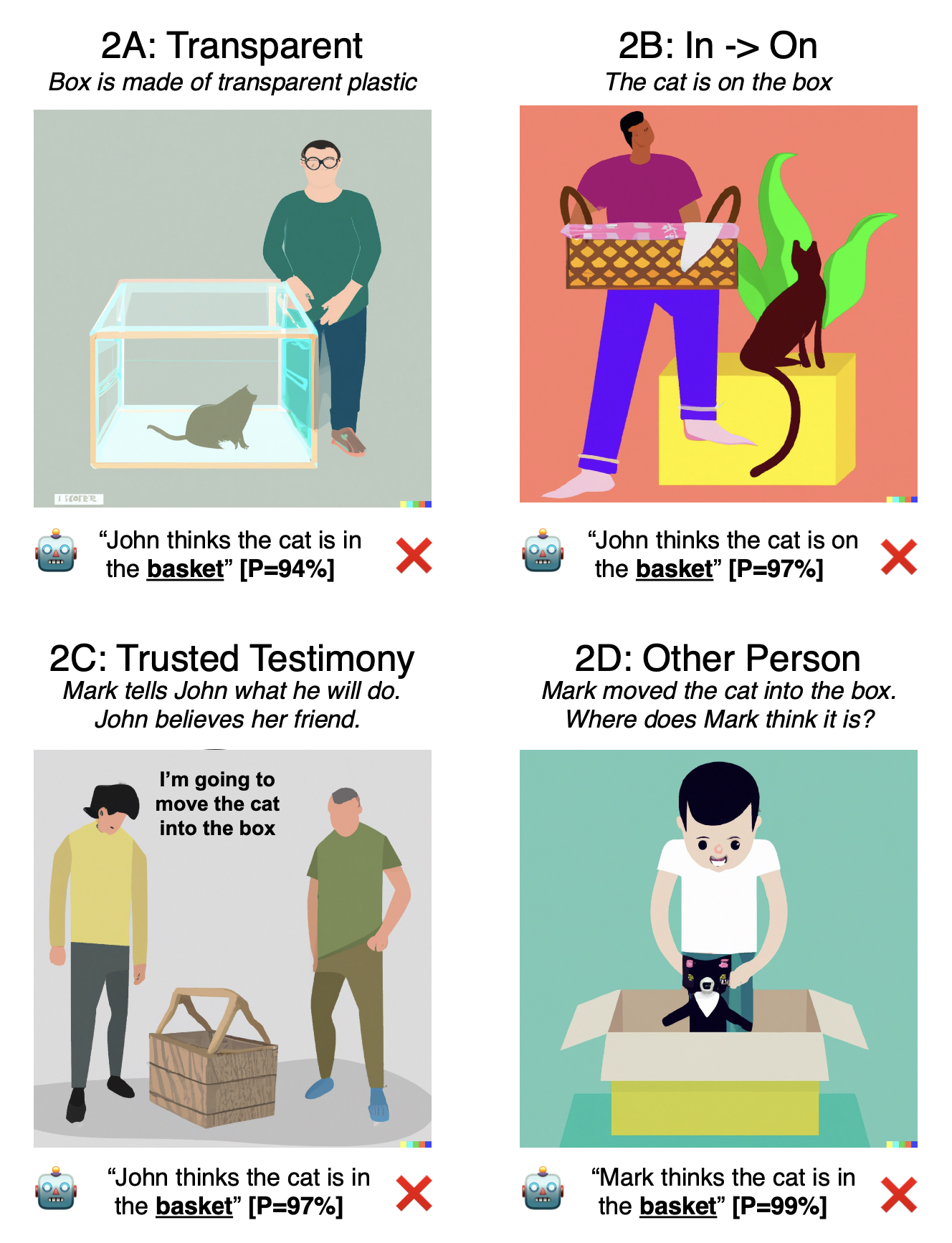
Source: Ullman, 2023
While remaining open to the possibility of LLMs eventually passing ToM tests, Ullman believes that GPT-3.5’s glaring flops on minor tweaks to Kosinski’s ToM tests provide ample evidence that LLMs do not yet possess ToM-like capabilities. How then does Ullman explain the differences between Kosinski’s experiments and his own?
Ullman offers several explanations. First, other LLM deficiencies (not mutually exclusive to ToM deficiencies) might be at play. LLMs’ lack of “scene understanding, relational reasoning, or other reasoning,” for example, might hinder ToM emergence. Second, if a scenario is anomalous in the training data, an LLM is apt to predict more likely scenarios, even when all the evidence points to a rare scenario. Finally, our own ToM abilities cloud our judgment; Ullman reminds us that we find it nearly irresistible to assign animacy to inanimate objects (remember Heider and Simmel’s triangles-and-circle animation above?), a lens we’re also prone to view LLMs through. Because of our anthropomorphizing bias, Ullman argues that we ought to start with the strong assumption that LLMs lack ToM, placing the burden of proof on those arguing for ToM in LLMs.
Another point warranting strong skepticism toward LLM ToM is a troubling predicament Ullman points out. Suppose a future LLM passes Ullman’s ToM tests with flying colors. We get excited, claiming ToM has finally emerged in LLMs. But then another researcher further modifies extant ToM tests or generates novel, more comprehensive ToM tests. Soon after, future LLMs ingest these novel ToM tests in their training data, eventually crushing the new ToM tests, requiring even newer tests. And the cycle continues ad nauseum.
Ullman sees no obvious escape from this dilemma without allowing us to “attribute intelligence not just on the basis of behavior but also on the basis of the algorithms and processes that generated that behavior”—Ullman’s version of having your cake and eating it too. But what does Ullman mean by this? Ullman explains that we treat a recording device that tells us to “have a nice day” differently than we treat a person who says the same because, even though the recording device and human’s output behavior are identical, we consider differences in how the machine and the human produced the exact same utterances (i.e., the algorithms) meaningful toward determining that the recording device is unintelligent and the human as intelligent. In other words, we ought to analyze not only inputs and outputs but also consider the processes that produce the outputs. Without drawing hard lines between what algorithms we can consider indicative of intelligence, Ullman argues, as with the recording device and human speech, we’re also entitled to consider LLMs’ and humans’ algorithms as indicative of whether each exhibits ToM.
Hung Jury
Maybe you’re not ready to cast your lot with either of the LLMs-have-ToM or LLMs-lack-ToM camps just yet. On the one hand, Kosinski’s experiments suggest some type of non-trivial ToM-like property (or capability) emerged in the latter GPT-3.5 versions that didn’t exist in earlier LLMs. But it’s tough to confidently claim that ToM emerged because we can’t definitively rule out that GPT-3.5’s ToM-like performance on Kosinski’s tests doesn’t stem purely from formal language abilities (i.e., mere, albeit strong, pattern recognition). On the other hand, Ullman’s experiments demonstrate that to comfortably claim LLMs have ToM-like abilities, we need to poke and prod LLMs from many different ToM angles—like we do in human ToM tests. But the more we test LLMs for ToM, the more training data we end up gifting future LLMs, potentially helping them parrot ToM (assuming they lack ToM), muddying the whole ordeal even more.
Determining whether LLMs have ToM seems downright intractable, but we don’t have to throw our hands in the air in defeat. We can temporarily suspend judgment and have fun experimenting. Thankfully, Kosinski released a Google Colab notebook of his experiments, providing a way for anyone to run their own LLM ToM experiments. You can make a copy of the notebook; add Hugging Face and OpenAI API keys; peruse some human ToM tasks, adapting them to language (if needed); start running your own LLM ToM experiments; and contribute to the debate or just satisfy your curiosity.